Google DeepMind unveiled Robotic Transformer 2 (RT-2) on Friday, marking a groundbreaking advancement in the field of robotics. This cutting-edge vision-language-action (VLA) model harnesses data collected from the vast expanse of the Internet to enhance robotic control via natural language instructions. The overarching objective is to engineer versatile robots capable of seamlessly navigating human environments, akin to iconic fictional robots like WALL-E or C-3PO.
In the quest for learning new tasks, humans frequently turn to reading and observation. RT-2 employs a formidable language model, reminiscent of the technology underpinning ChatGPT, extensively trained on textual and visual content sourced from the online domain. Leveraging this wealth of information, RT-2 adeptly discerns patterns and executes actions, even when it hasn’t received specialized training for those specific tasks—a remarkable feat known as generalization.
To illustrate this capability, Google illustrates how RT-2 empowers a robot to identify and dispose of refuse without undergoing dedicated training for this task. It leverages its comprehension of what constitutes trash and how it is conventionally discarded to navigate its actions. Remarkably, RT-2 can even recognize discarded food packaging or banana peels as trash, deftly handling potential ambiguities.
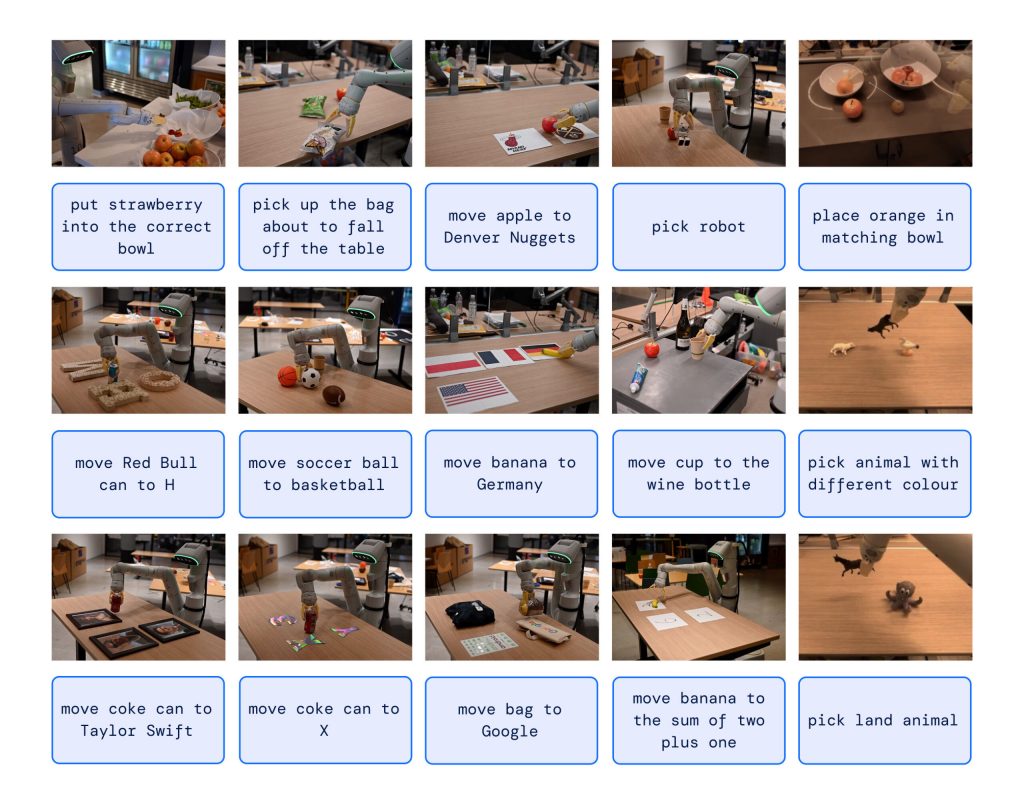
In a different instance, as reported by The New York Times, a Google engineer issued the command, “Retrieve the extinct creature,” prompting the RT-2 robot to successfully identify and select a dinosaur from a group of three figurines arranged on a table.
This particular ability stands out because traditional robot training has typically relied on an extensive dataset manually collected, a process made challenging by its high time and cost requirements to encompass every conceivable scenario. To put it simply, the real world is a constantly changing environment, with evolving situations and object configurations. An effective robotic assistant must possess the capacity to adapt in real-time, a task that is impossible to achieve through explicit programming alone, and this is precisely where RT-2 excels.
More than meets the eye
Google DeepMind has strategically harnessed the capabilities of transformer AI models with RT-2, capitalizing on their knack for information generalization. RT-2 builds upon earlier AI research at Google, drawing inspiration from the Pathways Language and Image model (PaLI-X) and the Pathways Language model Embodied (PaLM-E). Moreover, RT-2 underwent co-training with data from its predecessor model, RT-1, which was collected by 13 robots in an “office kitchen environment” over a 17-month period.
The architecture of RT-2 involves refining a pre-trained VLM model using robotics and web data. This refined model processes images from robot cameras and generates predictions for the actions the robot should undertake.
Given RT-2’s use of a language model for information processing, Google opted to represent actions as tokens, which are typically fragments of words. Google explained that to control a robot effectively, it must be trained to produce actions. They tackled this challenge by representing actions as tokens in the model’s output, akin to language tokens, and describing actions as strings that can be processed using standard natural language tokenization techniques.
In the development of RT-2, researchers employed the same method of breaking down robot actions into smaller components as they did with the initial version, RT-1. They discovered that by representing these actions as strings or codes, they could teach the robot new skills using the same learning models used for processing web data.
The model also incorporates chain-of-thought reasoning, allowing it to engage in multi-stage reasoning, such as selecting an alternative tool (e.g., using a rock as an improvised hammer) or choosing the best beverage for a fatigued individual (e.g., an energy drink).
Google reported that in more than 6,000 trials, RT-2 demonstrated performance on par with its predecessor, RT-1, for tasks it was trained on, referred to as “seen” tasks. However, when subjected to new, “unseen” scenarios, RT-2 exhibited a nearly doubled performance at 62 percent, compared to RT-1’s 32 percent.
While RT-2 showcases adaptability by applying its learned knowledge to novel situations, Google acknowledges its imperfections. In the “Limitations” section of the RT-2 technical paper, the researchers concede that including web data in the training material enhances generalization for semantic and visual concepts but does not magically imbue the robot with new physical motion abilities it hasn’t already acquired from its predecessor’s training data. In essence, it improves its utilization of actions it has already practiced in innovative ways.
Google DeepMind’s ultimate objective is to create versatile robots, but the company recognizes that substantial research lies ahead before reaching that goal. Nevertheless, technologies like RT-2 represent a substantial stride in that direction.
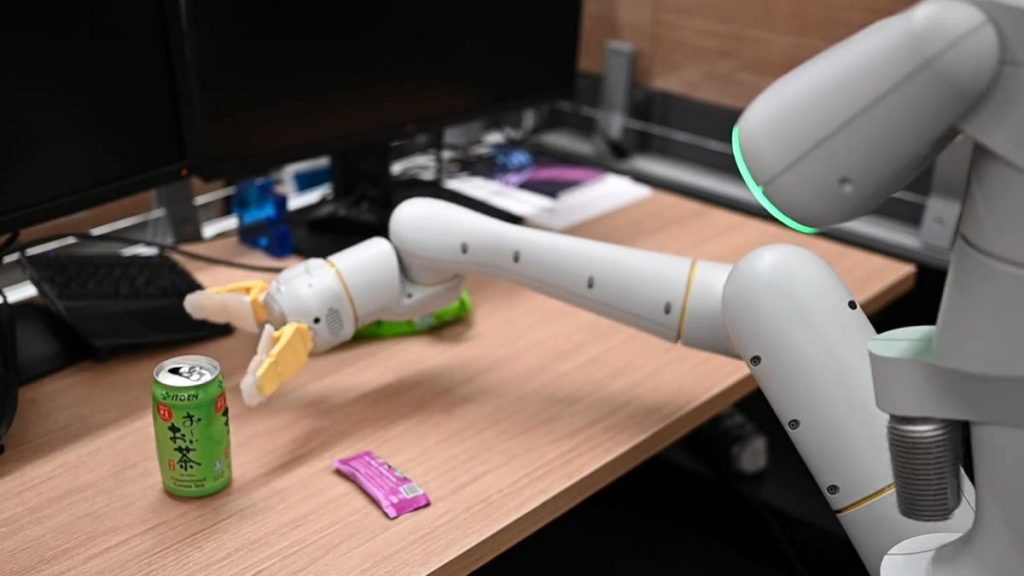